Contents
A Quick Guide to Model Monitoring Techniques
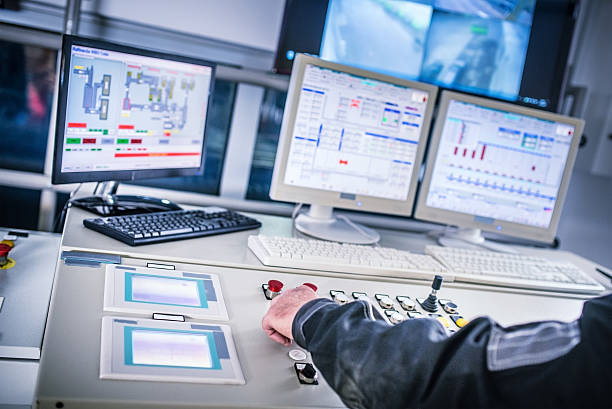
When you run a business in any field, keeping track of the processes of your business is one of the most crucial parts because it helps you maintain fluency in the processes and avoid any problems. With drastic growth and developments in technology and machine learning, people have started using ML models to monitor their business processes. They create a customized ML model that thoroughly analyses the data and reports accordingly.
There are various techniques that can help you monitor the performance of your ML models. You can refer to these points to strategize your ML model monitoring techniques:
Quick Guide on Model Monitoring Techniques
Ensure service health
When you use machine learning to monitor your data, you must check your ML model regularly to ensure the proper working of these models. If these ML models stop giving accurate results, they can misguide you into believing the wrong data analytics. Hence, it is important for you to get your ML model checked constantly.
Data quality
There are certain aspects of data that you must regularly check to ensure the quality of the data your ML model is engineering. These aspects include data distribution, range compliance, feature correlations and statistics, and other data behavior.
Drifts
There are chances that your data can experience drastic drifts because of external reasons like stock market fluctuations and changes in social dynamics. In such cases, it is important to indicate the reason for the fluctuations to avoid confusion in the future.
Track model performance
There are chances that your model is not giving accurate results because of specific errors. In such a case, you can test it with a different set of data so that you can detect the outliers or the error triggers. It can be subject to occasional fluctuations that don’t have any major effects. But it would be best if you looked out for regular decays in your model and changed them in time before they cause any problems.
Segment assessment
When you assess your model’s performance, it is advisable to analyze it in segments so that you can segregate the parts that work perfectly away from the parts that are exhibiting errors. This way, you will be able to point out the problem area and deal with it without any hassle.
Ensure accuracy
When the data is concerned with industries like healthcare, finance, and education, you must pay extra attention to the accuracy of the data reports because these reports can affect large-scale decisions in these industries. These industries directly affect people’s lives, so if the data that predicts information related to these industries is not accurate, it will also have irreversible effects on people’s lives.
Outliers
Models can make all kinds of errors, from minute errors to serious errors. Unless they lead to a complete model failure, these errors can be dealt with unless they lead to a complete model failure. You can design ways to handle these outliers that lead to errors. Sometimes it is advisable to create the solutions manually and not in an automated manner because some errors are beyond the understanding of Machine Learning and require human understanding to be dealt with.
The above-mentioned points are some of the techniques that can help you strategize the ML model monitoring techniques. You can find various companies that provide services that monitor your ML model and provide various solutions to deal with errors.